If Carlos Alcaraz is the prince of the drop shot, Alexander Bublik is the court jester. We learned this week that Bublik hits droppers more than any other tour regular, about once every 14 points. That’s three times as often as tour average. No one else goes to the well more than once per 19 points.
Persistence aside, Sasha’s results are mixed: He wins about 45% of those points. That’s unimpressive compared to the ATP norm of 54%, and it’s particularly weak next to Alcaraz’s mark of 62%. Assuming that drop shots are, on average, hit from a neutral rally position, one in which each player has a 50% chance of winning the point, Bublik costs himself 3.3 points per thousand with his drop shot. In the last decade, only Benoit Paire has been worse.
On the other hand, the number rests on a big assumption. Alcaraz excels from the baseline; Bublik relies more on his serve. For any given situation–say, 5th stroke of a second-serve point, ball coming to the backhand side–Carlitos probably has a better chance of winning it, drop shot or not. Indeed, based on Match Charting Project data, Alcaraz wins 52% of points from that position. Bublik manages only 46%.
That’s typical. Here are the six situations in which Bublik hits the most drop shots, broken down by whether he is the server or returner, whether it’s a first- or second-serve point, the stage of the rally, and whether he’s faced with a forehand- or backhand-side shot. The table shows the probability that he wins the point if he doesn’t hit a drop shot:
Sv/Ret Serve Shot Side Exp W% Sv 1st 3rd FH 57.4% Ret 2nd 4th BH 42.8% Sv 2nd 3rd FH 48.2% Sv 1st 3rd BH 51.6% Ret 2nd 4th FH 42.5% Sv 2nd 3rd BH 46.1% Ret 1st 6th+ FH 42.2%
Only two of these scenarios favor Sasha: Plus-one forehands and plus-one backhands behind a first serve. Just about anything else and he’s the underdog.
Here are the same six situations, with expected point winning percentages for Alcaraz:
Sv/Ret Serve Shot Side Exp W% Sv 1st 3rd FH 60.7% Ret 2nd 4th BH 51.5% Sv 2nd 3rd FH 57.3% Sv 1st 3rd BH 54.1% Ret 2nd 4th FH 53.6% Sv 2nd 3rd BH 50.7% Ret 1st 6th+ FH 55.1%
When Carlitos opts for a drop shot, he’s trading in what’s already a positive expectation for one that he hopes is even rosier.
Repeat this exercise for every situation in which Bublik has hit a drop shot, take a weighted average, and we find that had he not hit drop shots, he would have won 46.5% of those points. With that in mind, his 45.4% drop-shot winning percentage doesn’t look so bad.
The recalculation doesn’t tell us that Bublik’s drop shot is good, but it does make the tactic look more viable. We’re assuming that in the aggregate, all shot opportunities with the same profile (i.e. second-serve point, ball to the backhand for the fifth shot of the rally) are about the same. That’s just an approximation, so a gap of one percentage point could occur because Sasha chooses lower-percentage moments to hit the drop. There’s even a sliver of evidence that he does so: Eight of his charted drop shots are backhands on the seventh shot of the rally or later of his own first-serve points. Those sound like desperate efforts to finish a point he’s given up on, and sure enough, he lost all eight. Take those out of the equation, and his win percentage on drop shots is exactly the same as when he hits something else.
Drops in expectation
Go through the same exercise for every player, and the drop-shot leaderboard takes on a different look.
Some players, like Kei Nishikori and Nicolas Jarry, win a very high percentage of drop shot points and exceed expectations by a wide margin. Others, like Alcaraz, see less of a benefit from their drop shot, in part because their other options are so good. Still others, like Daniil Medvedev, win more than half of drop-shot points, but because of the rest of their game and the moments they choose to deploy the drop, they may be sacrificing some points when they do so.
Call the new stat Drop Shot Wins Over Expectation, or DSWOE: the ratio of drop-shot success rate to non-drop-shot winning percentage, taking into account the situations in which the player chooses the drop.
Among the 60 players with the most charted points since 2015, here’s the top of the list–the men who gain the most per drop shot–along with a few notable names in Bublik’s section of the list, plus the most extreme laggards:
Player Drop W% Exp W% DSWOE Nicolas Jarry 65.3% 50.4% 1.30 Lucas Pouille 60.3% 48.1% 1.25 Kei Nishikori 68.1% 54.5% 1.25 Sebastian Baez 63.2% 50.9% 1.24 Richard Gasquet 60.7% 50.0% 1.22 Kevin Anderson 53.8% 44.6% 1.21 Reilly Opelka 52.1% 43.5% 1.20 Marton Fucsovics 58.2% 49.5% 1.18 Alejandro Davidovich Fokina 59.3% 50.7% 1.17 Roger Federer 59.5% 51.4% 1.16 Robin Haase 54.7% 47.8% 1.14 Frances Tiafoe 54.6% 48.0% 1.14 Pablo Carreno Busta 58.9% 52.2% 1.13 Dominic Thiem 57.1% 50.7% 1.13 Carlos Alcaraz 62.1% 55.7% 1.12 Rafael Nadal 61.5% 55.4% 1.11 Andy Murray 55.7% 50.5% 1.10 … Holger Rune 51.4% 51.3% 1.00 Grigor Dimitrov 47.7% 47.9% 0.99 Alexander Bublik 45.4% 46.5% 0.98 Daniil Medvedev 53.0% 54.8% 0.97 Novak Djokovic 50.8% 52.9% 0.96 … Stan Wawrinka 45.3% 48.9% 0.93 Milos Raonic 38.0% 41.3% 0.92 Benoit Paire 42.9% 46.8% 0.92 Tommy Paul 47.0% 51.5% 0.91 Aslan Karatsev 39.0% 49.9% 0.70
Surrounded by names like Rune and Djokovic, Bublik doesn’t seem so bad. Alcaraz, on the other hand, doesn’t stand out as much. He and list-neighbor Rafael Nadal are outrageously good in rallies whether they hit a drop shot or not. Even a world-class drop shot is only so much better than a standard Rafa or Alcaraz topspin groundstroke.
Tour average is around 1.05, meaning that the typical player does a bit better when they hit a drop shot than they would have had they chosen a different shot in the same situation. That tells us something that we probably suspected: Players are generally good at choosing the right moment to unleash the drop.
With this more fine-grained notion of expectations, we can re-calculate the number of points per thousand that each player gains or loses from drop shots. It is a function of both success rate (relative to expectations) and frequency. Nishikori and Jarry get great results from the drop but employ it rarely; men like Alcaraz and Sebastian Baez gain more points overall because they hit droppers so much more often.
Here are the players who gain the most points, along with the five tour regulars at the bottom of the list:
Player Freq% W% - Exp% DPOE/1000 Sebastian Baez 3.9% 12.3% 4.8 Alejandro Davidovich Fokina 5.2% 8.5% 4.5 Lucas Pouille 2.9% 12.2% 3.5 Carlos Alcaraz 5.4% 6.4% 3.4 Richard Gasquet 2.8% 10.8% 3.0 Robin Haase 3.9% 6.9% 2.7 Kei Nishikori 2.0% 13.6% 2.7 Frances Tiafoe 3.2% 6.6% 2.1 Pablo Carreno Busta 2.8% 6.7% 1.9 Nicolas Jarry 1.2% 14.9% 1.8 Fabio Fognini 3.7% 4.7% 1.8 Andy Murray 3.3% 5.2% 1.7 Dominic Thiem 2.6% 6.4% 1.7 Marton Fucsovics 1.9% 8.7% 1.7 Roger Federer 2.0% 8.1% 1.6 … Novak Djokovic 3.3% -2.1% -0.7 Alexander Bublik 7.2% -1.0% -0.8 Lorenzo Musetti 5.1% -2.3% -1.2 Aslan Karatsev 1.2% -10.9% -1.3 Benoit Paire 5.4% -3.9% -2.1
Five (or 4.8) points per thousand might not sound like a lot, but it represents the difference between Baez having a place in the top 20 and residing well outside of it. Alcaraz still grades well here, if not as much as he did before making all of the adjustments. Bublik scores closer to neutral too. His drop shot is probably more useful for earning him highlight-reel screentime than it is for winning points, but it isn’t hurting him that much.
Side matters
Armed with these adjustments, we can compare each player’s forehand and backhand drop shots, as well. Bublik has a fairly wide split. He wins just over 50% of points when he hits a forehand drop shot, next to only 39% behind a backhand drop shot. His expectations when faced with a backhand are worse in general, but not that much worse. His forehand drop shot success rate is two percentage points better than if he went with a standard groundstroke, while his backhand drop shot is five points worse.
So Sasha, if you’re reading this: We all love your drop shots. But maybe take it easy with the backhands.
The best forehand drop shots, compared to how the player would have fared with a different shot, belong(ed) to Kevin Anderson, Sebastian Baez, Lucas Pouille, Marton Fucsovics, and Nishikori, with Roger Federer not far behind. The most effective backhand droppers are those of Jarry, Reilly Opelka, Pouille, John Isner, and Richard Gasquet. “Expectations” is the key word for Opelka and Isner: They didn’t win a lot of points once a rally was underway, so a moderately good drop scores very well by comparison.
Here is the field of 60 regulars from the last decade. As usual, top right is good, bottom left is… yikes, Aslan Karatsev.
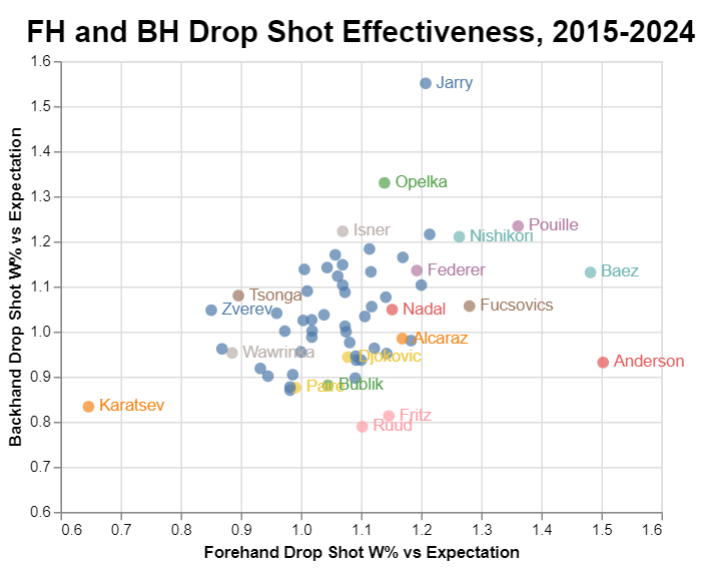
There are innumerable way to divide these numbers even further, and I know you’re tempted. But with drop shots, there is only so much data. Some of the outliers here, like Jarry and Anderson, are probably a bit aided by luck. Men who don’t hit many drop shots might only have a few dozen attempts on their weaker side. The standouts probably are better than average, but limits of our data lead us to overstate their advantage.
At least with the forehand/backhand division, adjusted for how players would have fared with something other than a drop shot, we can get some hints as to how our faves can improve their games. Taylor Fritz has a strong backhand, and I doubt the points he’s losing with his backhand drop shot are making it any more effective. Alexander Zverev isn’t doing himself any favors with his occasional forehand droppers. Karatsev, well… not everyone can excel at everything.
Bublik, despite his negative numbers in the aggregate, has an effective forehand drop shot. With the power of his serve and forehand, he’ll continue to earn plenty of opportunities to use it. If he resists the urge to showboat on his backhand side, the court jester of the drop shot could continue to show off his touch and still earn a more coveted position in the tactic’s royal house.
* * *
Subscribe to the blog to receive each new post by email: